Institutional Analytics: Big Brother on Campus?
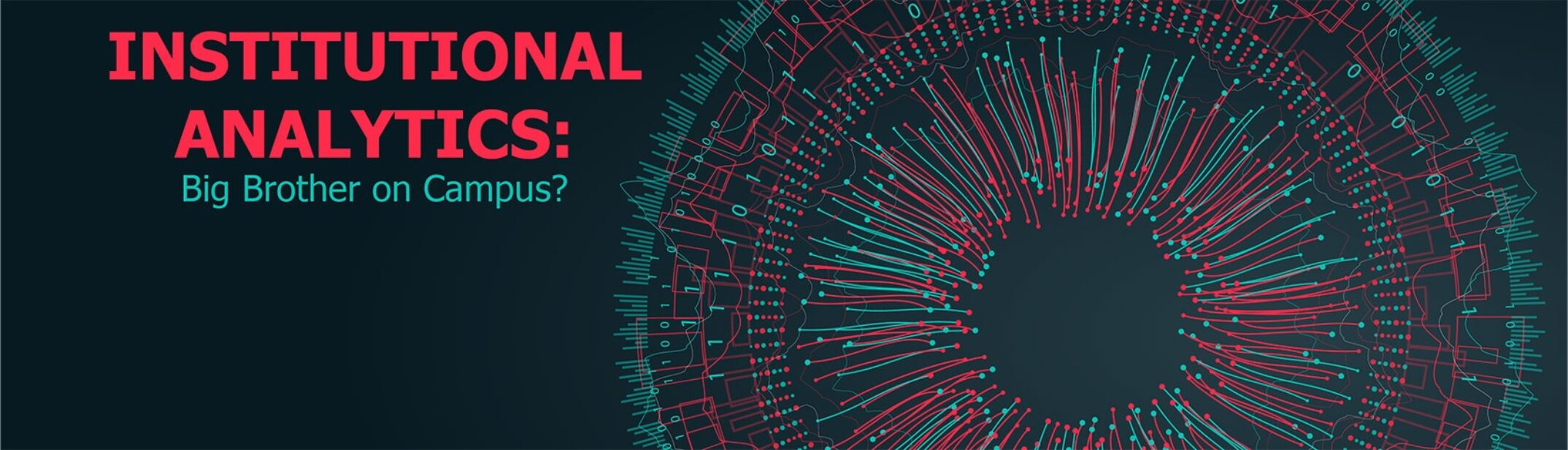
George Orwell’s classic 1984 is a staple in high school curriculum across the country. And while this dystopian world is merely discussed in those classrooms, Big Brother is alive and watching college students across the country through the use of big data and analytics. The tracking of information is not a new practice; institutions have been using test scores and student performance even before the digital age to gain insights and adjust curriculum to better serve learners.
The amount of data being collected on today’s campuses is staggering. Where do students spend time? Who do they spend time with? How do they answer test questions? What do they prefer to eat? Individually, these data points may seems relatively benign. But when aggregated, analyzed, and ultimately compared, they can provide very specific insights about student activity and even predict behaviors. Campuses are exploring and finding new ways to look at the data, and the potential to use it to shape student behavior, increase academic success, and impact student well-being.
This practice is double-edged by nature. We want institutions to grow and adapt, in order to better serve students. But with that comes a significant cost, which cannot be ignored: privacy.
Data Obsessed
The pursuit of personal growth is a very real trend within our society today. As a result, we have become obsessed with tracking our own personal metadata. Tracking what we eat; how many steps we take in a day; how we utilize our time. Software can then compare and analyze this data, drawing inferences and recommending ways to improve. However, the apps we use may be saving our data and using this information in ways we are unaware of. Yet tracking our own data feels like a private effort; even though there might be other implications it doesn’t feel like a violation, so we gladly check the “I accept terms” box. That is, until a week later we get notified that our favorite products are on sale at our local store based on our purchase history. Or we start to see ads on random websites for items that we decided we just weren’t quite ready to buy. Then, our privacy might feel violated.
While many giant corporations continue to capitalize on our data for purely monetary reasons, why is there sensitivity surrounding the idea of higher education institutions using data to improve operational needs, academic performance, and student safety?
Important Terms
Big Data: Extremely large data sets that may be analyzed computationally to reveal patterns, trends, and associations, especially relating to human behavior and interactions.
Descriptive Analytics: Summarizes data from the past. What happened?
Institutional Analytics: Involves high-order institutional metrics like attrition rates or year-to-year completion rates.
Learning Analytics: Focused on the individual learner and provides actionable information to make decisions in a given course or set of courses.
Predictive Analytics: Analyzing what has happened and predicting the likelihood something will happen in the future. What might happen?
Prescriptive Analytics: Analyze a number of different outcomes and advise a solution. What will happen, and why?
Data on Campus
One university is currently uses 800 data points to generate lists of students likely to drop out.1 These data points include, but are not limited to, student demographic information, financial information, and their location on campus in relationship to things like vending machines, libraries, residence halls, and the student union center. By using sophisticated algorithms, campuses now have the capability to improve operational needs, gauge teacher effectiveness, target struggling students, and flag at-risk behaviors. While there are a multitude of different types of data that institutions are collecting, not all data is equal. Consider the following:
Operational and Logistical Needs
The use of student statistics anonymously to improve institutional efficiencies and better understand the student population needs may be the most benign use of data. Determining what types of meals to serve in dining halls by analyzing popular menu items, or setting library operating hours based on when and how long students are in the building, can help institutions better serve their student populations. And since it’s tracking trends, not individual students, there’s a level of anonymity associated with it.
Adaptive Courseware
Some universities are using learning analytics and adaptive courseware to evaluate the effectiveness of learning tools, assess teaching pedagogies, and even target where a student or entire class might be struggling. Adaptive courseware can be used to monitor student performance and track behaviors. How long did it take for a student to answer a question? In what areas of curriculum did a student struggle? Metadata is collected and compared, notifying a professor when a student might need additional support, and in what areas. These interventions could possibly be the difference between success and failure for some students and greatly impact academic outcomes and institutional effectiveness.
Campus Safety
Believe it or not, your student ID card is much more than a crappy photo. It can also double as a monitoring device, tracking where you are and where you have been. As you walk into a building or scan into a room, your institution can see when you arrived to class and who you are with. If you live on campus, they can track what time you leave and return home. Colleges and universities can use this information to better understand campus activity and assign resources appropriately, influencing hours of operation and staffing schedules. It could also be used to understand where students are late at night and modify how campus security operates, to ensure a higher level of student safety.
This example really begins to toe the line on potential ethical issues. If the data shows a building on campus is heavily used for late-night study groups, we feel better about the institution identifying this trend and increasing security in this area. However, if this same data is used to identify a particular group of students that habitually returns home every Thursday from a late night out, it begins to feel like an infringement of privacy.
At-Risk Student Behavior
Mental health issues are a major concern on college campuses across the country. For many freshman, starting college is an overwhelming number of first time experiences; the academic workload, a new social situation, and an unfamiliar physical environment can be very hard to grapple with. A recent survey by the American College of Health Association (ACHA) found that 40% of students reported that they have felt so depressed in the prior year that it was difficult for them to function. The three top factors that students reported affected their individual academic performance were: Stress 31.7%, Anxiety 25.1%, and Depression 16.8%.2
What if data was used to identify behavioral trends, in an effort to flag at-risk students? Information could detect a student who hasn’t attended class in a week, has been absent from their typical social events, hasn’t visited any campus resources, and whose dining hall activity indicates they are only eating once a day. If these points of data were compared, they could identify alarming behaviors or sudden changes to routines. The use of predictive analytics could then alert counselors or student support staff of potential concern, triggering interventions that could prevent dropouts, unhealthy behaviors, or suicide.
Behavioral and Institutional Norms
What happens when data is tracked and connected to behaviors which are outside of the institutional-prescribed norms? For instance, what if the student data a university collected was used to evaluate behaviors and trigger mandatory curriculum changes such as a physical education class, tutoring sessions, or increased counselor visits? At this point, the student no longer has the freedom to choose and behaviors that are deemed unhealthy are at the discretion of the institution’s administration.
Your Digital Fingerprint
The science and sophistication of predictive and prescriptive analytics is growing. Some institutions already have the ability to predict success rates and GPAs of their incoming freshman class based on demographic information and learning habits, such as how long it takes them to complete their homework. Could this collection of data points become a new digital fingerprint of the future that follows you the rest of your life? What if graduate programs and potential employers begin asking for more than just transcripts? What if they also want access to all of the data that has been tracked and associated with our behaviors?
Ethical Debate
Harnessing the full capability of data can lead to some clear ethical issues that need to be addressed within institutions, especially when that data goes from anonymous to personal. There is also a difference between prediction and prescription: using data to determine how to best serve students versus deliberately tracking, categorizing, and defining a student’s academic abilities. On one hand, data is used to promote student success. On the other, students are profiled and prescribed academic achievement ratings. How easily is that line crossed? Do institutions have the right to do so? Does this violate a student’s right to privacy?
The 1974 Family Education Rights and Privacy Act (FERPA) guarantees privacy to student “education records.”3 However, it was enacted when records were perceived only in written form. With only outdated legislation, there is debate whether student metadata is protection under FERPA, as well as what is considered an “educational record.” The conversation has already started regarding PK-12 student privacy. Washington State legislators are attempting to safeguard student data with the Super Act. Other states are starting to enact legislation regarding student privacy in higher education, however, it is a state by state case. Perhaps it is only a matter of time before FERPA is amended and additional privacy regulation will extend to students on college campuses. Currently, colleges and universities appear to be using this type of data responsibly, applying it with the sole purpose of positively impacting academic outcomes and better supporting the success of their students. Appropriate measures are being employed to safeguard this information and maintain student anonymity.
The Power to Affect Design
What if architects could use institutional analytics to inform design? What if this information could help calibrate and effectively create student spaces and residence halls to better support the whole student? Due to the nature of the design and construction process, architecture often experiences a slow feedback loop. Once a project’s design is completed, it is sometimes not occupied until years later. Because of this, it can be challenging to truly know what design goals excelled, and which didn't quite hit the mark. The deliberate use of data could provide another tool in our design toolkit, helping us interpret and complement the input of user groups based on proven analytics.
In the future, designers could have the ability to collaborate with higher education institutions to tune design decisions with some of the data that is currently being tracked on campuses. Currently, decisions such as site selection and programmatic amenities are based primarily on evidence that is often subjective and circumstantial. However, being able to harness and apply imperial data relative to how people actually inhabit and utilize buildings could reveal surprising correlations and new ways of thinking about the built environment. This data could inform the programming phase of a project, allowing designers to better understand campuses in more finite detail. Analyzing trends of where students prefer to study or spend free time could help determine what types of amenities should be provided based on how they are utilized. Discovering the nuances of colleges and universities and their individualized student populations could give architects a greater ability to design for what is truly needed, creating a potential for a greater return on an institution’s investment. The more information we have, the more impactful our designs will be.
Conclusion
According to a KPMG Survey (2015) only 41% of senior administrator respondents were using student data for predictive analytics, and just 29% reported having the internal capacity to analyze their own student data.4 While there is a myriad of logistics that institutions will have to overcome, as well as the serious ethical debate over student privacy, the potential benefits of data-analytics could be dramatic for our nation’s institutions and potentially life-changing for students. The possibilities of using institutional data to positively impact overall student wellness and help universities improve inefficiencies are a reality. It is no secret that Big Brother is already watching, keeping track of the products we buy, the restaurants we visit, or how many calories we burn each week. The question isn’t if data analytics can impact higher education. It’s how.
References
1 Wang, A. X. (2018, March 13). A US university is tracking students' locations to predict future dropouts. Retrieved October 14, 2018, from https://qz.com/1226474/ a-us-university-is-tracking-students-locations-to-predict-future-dropouts/
2 National College Health Assessment. (2017). Fall 2017 Reference Group Executive Summary [Brochure]. Retrieved Oct 14, 2018, from https://www.acha.org/documents/ncha/ NCHA-II_FALL_2017_REFERENCE_GROUP_ EXECUTIVE_SUMMARY.pdf
3 U.S. Department of Education. (2018). Family Educational Rights and Privacy Act (FERPA). Retrieved October 14, 2018, from https://www2.ed.gov/policy/ gen/guid/fpco/ferpa/index.html
4 KPMG Higher Education. (2015). 2015–2016 Higher Education Industry Outlook Survey [Brochure]. DE. Retrieved October 14, 2018, from https://assets.kpmg.com/content/ dam/kpmg/pdf/2016/06/ co-gv-4-2015-2016-higher-education-industry-outlook-survey.pdf
Additional Resources
Kurzweil, M., & Stevens, M. (2018, May 7). Setting the Table: Responsible Use of Student Data in Higher Education. Retrieved October 14, 2018, from https://er.educause.edu/articles/2018/5/ setting-the-table-responsible-use-of-student-data-in-higher-education
Pelletier, S. G. (2015, December 10). Taming "Big Data": Using Data Analytics for Student Success and Institutional Intelligence. Retrieved October 14, 2018, from https://www.agb.org/trusteeship/2015/ taming-big-data-using-data-analytics-for-student-success-and-institutional